Beiträge
Themengruppen
Recherche
Service
- Was ist Wissensmangement?
- Open Journal of Knowledge Management
- Artikel-Guidelines
- Newsletter
- Kalender
- Wissensmanagement-Anbieter
- Partner
- Mediadaten
Community
Sponsoren
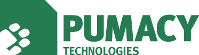
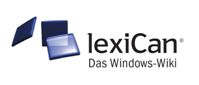
Knitting the kNet - Towards a Global Net of Knowledge
29. März 2010 von Dr. Heiko HallerkNet is the vision of a future knowledge-sharing platform. In a fictitious scenario, this article describes how certain semantic Internet services could support scientific knowledge sharing and leverage knowledge transfer. In the following discussion, the core features of this platform are discussed and compared with existing state of the art systems.
This paper was published in the Open Journal of Knowledge Management, I/2010.
The Scenario
Sylvia is a student. She wants to find out about the xy diet that has been recommended to her by a friend. This new diet stipulates not combining certain kinds of food. To that end, Sylvia searches the kNet for evidence on xy diet’s effectiveness.
kNet is a heterogeneous distributed online knowledge system based on a common semantic modeling scheme. Sylvia queries kNet about the claim that xy diet is beneficial. The results reveal that over all, more than 10.000 people support this claim while less than 2.000 refute it. After she filters these claims down to those who provide trustable evidence, few are left which claim to have systematically tested out xy diet, but none of these studies are backed by bodies that fulfill her “scientific grade” trust preset (which currently is: either international university professors or institutions certified by a European government). So, Sylvia sets up a trigger to get notified of any such stronger evidence.
Bob is a PhD student in biochemistry. In a lab study, he finds out that a certain enzyme that some people lack might play a crucial role in the digestion of mixed diet. He writes a paper on this research and, along with a standard semantic model that outlines his findings on two levels of granularity, he submits it to his favorite kNet portal.
Xu Xie is a professor of life sciences. He gets notified of Bob’s paper because he has subscribed to cutting edge research reports in the field of inter-individual nutritional ergonomics. Xu reviews Bob’s paper and backs his claim as a referee.
According to the seminal article “kNitting the kNet”, that first described the idea of kNet in an upcoming online journal, the community of knowledge-engineers that dedicate themselves to cultivating the kNet by interweaving topics and carefully constructing overarching knowledge models, call themselves “kNitters”. Gunther is such a kNitter. He gets notified of Bob’s findings because he monitors upcoming basic research in the field of nutrition and now that Xu has backed Bob’s contribution, it shows up on Gunther’s list. He links Bob's results to the claim that xy diet is beneficial.
This is enough to trigger a notification to Sylvia, since now there is a first scientific indication that there might be something to the idea of xy diet. She quickly crosschecks the enzyme Bob described with her genome: She actually belongs to the group of people who lack the enzyme. After checking the chain of trust for Bob’s conclusion, she decides to give xy diet a try. After two months she has more energy and feels so much better, that she goes back to Bob’s model in kNet and confirms his claim by backing it with her personal experience. To support her claim, she configures her certified genomic services provider to open her respective genetic sequence to the public and link it to the online identity she has used in her kNet comment.
Sue, a nutritional researcher who has also noticed Bob’s research, evaluates Sylvia’s and other public responses to Bob’s findings. Thereby she finds out that while xy diet appears to be useless for most people, it is actually beneficial for those with a certain combination of genotypes. With these new findings, she writes a well-received survey paper in which she credits Bob’s research.
Meanwhile, Bob has earned enough scientific merits to get him a cumulative dissertation.
Discussion
Today – in early 2010 – kNet does not yet exist. It is a vision of how e-science could look like in a couple of years. Most of the technologies needed are already there – however a) they are not widely used and b) they are not yet sufficiently integrated with one another. To get a better idea what is behind the different aspects touched in this scenario, let us take a closer look:
When Sylvia first queries kNet about that diet’s claim, this is different from what we use today: She neither searches for one document that contains the right keywords (like nowadays’ web search), nor does she search for a simple fact or statement that is either present in a knowledge base or not (like it is supported by today’s semantic knowledge bases such as freebase [i], DBpedia[ii] or Semantic MediaWiki[iii]). Instead, she searches for meta-information about such a single statement – in this case how many people make or support that claim and how many refute it. From today’s state of the art, mainly two additional things are needed: a) Models and tools that allow to express opinions in a way that they can get aggregated and compared over large numbers. So, additional to the perspective of the primary semantic layer that models the actual facts and claims, there needs to be a secondary one that models meta-information about them, such as provenance, trustability, redundancy etc. b) Ways to aggregate semantic models from distributed sources in such a flexible manner that they can be sophistically filtered according to these meta-information on the fly. The ‘Linked Open Data’ community[iv] is working into this direction but neither of these two aspects is solved to date.
The reason why this is crucial becomes evident, when Sylvia filters down the large number of opinions first to those who provide evidence and then to those whose evidence she is willing to trust. Depending on the kinds of questions, there will be quite different criteria of whose judgment to trust. And users should be able to specify their own trust settings according to personal preference. Sylvia, e.g., has used her “scientific grade” trust preset which is formulated as either international university professors or institutions certified by a European government. Other such presets could be “accepted domain expert” which might be configured as persons with at least 95% positive trust ratings by identified users in the respective domain or simply people that I trust personally and those that they trust. For that latter example, as discussed below, users must also be able to publish their trust ratings, so other people can reuse them when calculating aggregated transitive trust ratings.
When Bob publishes his paper, it is first of all a regular research paper like we know it. If he would submit it to a classical scientific journal today, this would typically deprive him of the right to further distribute his own work. Open access[v] journals are an already existing better alternative here. However, his work would still have to pass the threshold of being simultaneously accepted by three experts or remain unpublished. When Bob submits his work to a kNet portal, at first, his publication has the status like an unrefereed technical report. With kNet, it would be the individual user who sets the thresholds of visibility when she is searching, not a community of experts (who might e.g. suppress work conflicting with their own approaches) setting the threshold before publication. In kNet, it would always be possible to publish – like it is today e.g. in a weblog. The difference lies in the surrounding framework that allows others, including domain experts, to find such publications based on the meaning of its content and to comment and rate each publication in a trustable and flexible way. For that, the framework would need to allow for verifiable identities and modeling the trust ratings between them. Then it would be possible that publications are first read and rated by those with low thresholds and high interest in the specific topic, and then by and by bubble up into the visibility of a larger public and higher level experts until it earns enough merits to reach the status of today’s scientific journal publications.
Today, “Liquid Democracy” systems like LiquidFeedback[vi] already allow to express one’s trust in someone else and even to restrict this trust to specific topics. This trust is propagated through the network of people and cumulates to those that justifiably (and revocably) represent the opinions of their supporters. However this paradigm of delegated voting is mainly designed for delegation of decision power and not for rating products or knowledge artifacts like articles. Some product rating systems like e.g. Epinions[vii] also already use some web-of-trust[viii] principles, and others like Revyu[ix] allow anyone to rate anything. However none of them combines all features required for the functionality described in the scenario. Most lack the facility to rate different aspects of an item and none of them integrates semantic search:
To be found based on its actual meaning, Bob’s publication needs to be augmented with a semantic model. This model would need to address the following perspectives: a) To contribute to a larger collaboratively constructed knowledge model, Bob’s model needs not only to indicate the topic, but represent the actual findings themselves (i.e. the finding that the presence of enzyme z facilitates the combined digestion of x and y, which otherwise can both be digested without enzyme z but only when consumed separately). This would happen in an adequate domain ontology of which there might be several competing ones, as the community struggles to find a common model. b) To be easily found by interested readers, the domain and kind of contribution would need to be represented in a more widely accepted modeling scheme. An existing system to annotate scientific articles on several semantic layers is e.g. SALT[x], which however is not widely used. Also, formal semantic domain models (ontologies) are still missing for many scientific domains, as are interdisciplinary higher-level ontologies that connect them.
A functionality that reappears throughout the scenario is the ability to set up subscriptions or triggers that actively notify a user when certain query results change or appear for the first time. This can be seen like a mixture of Google alerts[xi] (that work for all the sites indexed by Google but are restricted to mere keyword searches) and Semantic MediaWiki’s RSS result format[xii] (that allows to subscribe to the results of a semantic query but are restricted to the information stored in one single site).
A part of the scenario, that might seem futuristic to some people, is, when Sylvia checks her own genome for the ability to produce that enzyme. In fact this is already possible today with service providers like 23andMe[xiii]. Also, with SNPedia[xiv], a large collaborative semantically annotated database of such genetical information is already available.
Sylvia’s reporting her personal experience back into kNet in a verifiable manner illustrates how such a system could leverage a wave of research with large numbers of subjects that are much more easily available than in most current research settings.
The community of “kNitters” that is crucial to a platform like kNet, are the wikipedians and scientific bloggers of today: these thousands of people that dedicate some of their time to collaboratively interlinking bits of knowledge, refining the structure of public knowledge models and building bridges between domains. The title “Knitting the kNet” is of course homage to Sir Tim Berners-Lee, inventor of the World Wide Web and great visionary of the Semantic Web, who wrote a book about the web’s history and possible future entitled “Weaving the Web”[xv].
References
[iii] http://semantic-mediawiki.org/
[v] http://en.wikipedia.org/wiki/Open_access_(publishing)
[vi] http://liquidfeedback.org/
[vii] http://www.epinions.com/
[viii] http://en.wikipedia.org/wiki/Web_of_trust
[x] http://salt.semanticauthoring.org/
[xi] http://www.google.com/alerts
[xii] http://semantic-mediawiki.org/wiki/Help:RSS_format
Kommentare
Das Kommentarsystem ist zurzeit deaktiviert.
Schlagworte
Dieser Beitrag ist den folgenden Schlagworten zugeordnet