Beiträge
Themengruppen
Recherche
Service
- Was ist Wissensmangement?
- Open Journal of Knowledge Management
- Artikel-Guidelines
- Newsletter
- Kalender
- Wissensmanagement-Anbieter
- Partner
- Mediadaten
Community
Sponsoren
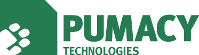
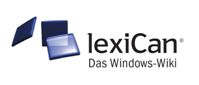
Social Forecasting for New Products
27. Juni 2012 von Aleksandar IvanovForecasting new products is hard for a retailer like Tchibo. There is no past data to use in statistical models, consumer surveys and test sales are expensive. Tchibo decided to apply Social Forecasting - a new approach from the Enterprise 2.0 area. Social Forecasting utilizes the knowledge of employees and transforms it into hard numbers, e.g. sales forecasts for new products. With Social Forecasting Tchibo was able to achieve extreme cost savings while maintaining a high accuracy in forecasting demand for new products.
This articel was also published in Open Journal of Knowledge Management, Issue V/2012.
The challenge: "Every week a new world"
Forecasting new products is extremely difficult. Tchibo is a retailer which introduces new products every week! With 2,500+ stores across Europe Tchibo is specialized on selling private-label clothing, household items, and consumer electronics. The company was founded in 1949 and was originally focused on coffee specialties which are still part of its business. Nowadays, Tchibo has evolved into a versatile retailer with exciting new product offerings.
Besides its regular product lines the company is introducing a small set of new products every week for a limited time! This is Tchibo’s signature approach for which Tchibo is known and which helps keeping customers coming back to Tchibo stores regularly.
The challenge with this approach is that it creates large planning and forecasting costs. Every week Tchibo must decide on the new products and the quantities to order from manufacturers. This planning process involves internal discussions as well as weekly test sales across dozens of stores, and weekly consumer surveys (Fig. 1).
Fig. 1: Forecasting process for new products at Tchibo before the use of Social Forecasting (Source: Author)
This process had a good forecasting accuracy for new products but the total costs of these activities amount to millions of Euro each year. Therefore management was looking for a way to reduce these costs while maintaining the forecasting accuracy for new products.
At that point Tchibo contacted CrowdWorx, a Social Forecasting vendor, to evaluate Social Forecasting in its business process.
The approach: Social Forecasting
In forecasting the most common approaches are surveys, expert judgment and data-driven statistical models (Armstrong, 2001). In recent years amidst the rise of Crowdsourcing and Web 2.0 a new Enterprise forecasting approach has emerged – Social Forecasting.
As the name implies, Social Forecasting is utilizing the knowledge of many people for forecasting. Unlike Crowdsourcing, Social Forecasting is focused on internal sources of knowledge, i.e. the knowledge of employees, not consumers. Unlike surveys, in Social Forecasting people are not paid for mere participation, but for accurate responses. This incentive mechanism improves the quality of responses (Ivanov, 2011). Figure. 2 visualizes the constituent elements of Social Forecasting.
Fig. 2: Elements of Social Forecasting (Source: Author)
In contrast to traditional Enterprise 2.0 approaches Social Forecasting does not generate thousands of comments or blog articles but actual numbers from employee knowledge. A special algorithm converts the opinions of all employees into one number. In this case Social Forecasting provided forecasted unit sales of each new product.
To achieve the best possible forecasting accuracy, Tchibo invited a cross-section of employees who have relevant knowledge about the market, the consumers, the competitors, and new trends. This forecasting crowd included shop managers, workers from distribution hubs, as well as various experts from headquarters. All these different people would contribute their knowledge into a Social Forecasting platform in the Tchibo Intranet.
Within a few weeks, Tchibo set up its CrowdWorx Social Forecasting platform and designed a communication approach towards its employees to achieve buy in. A key ingredient of the communication strategy was the fact that employees would now be able to give their opinions on highly relevant business topics (estimate the sales of new products). Since Tchibo was asking its employees for help on a real business issue this signaled that Tchibo values and appreciates the knowledge of its employees.
In addition to this theme of appreciation, Tchibo also introduced an element of competition by using rankings which would show the weekly top forecasters from across the organization.
Finally, some small material incentives were added in the form of Amazon vouchers which would reward the monthly top forecasters.
Once these measure were in place, the Social Forecasting process was launched. Each week shop managers, warehouse workers and employees from HQ visited the Social Forecasting portal and made their sales predictions for the upcoming new products (see Fig. 3).
Fig. 3: Forecasting process for new products with Social Forecasting (Source: Author)
The predictions from the crowd where available immediately after the closing of the forecast. No market research or test sales were required to derive these forecasts. After an initial ramp-up phase the actual sales figures started to arrive each week. These were entered into the system which then automatically calculated the top forecasters – those whose forecasts were closest to the actual sales figures. This gave a boost to participation and was very well received by employees (Preller and Rudzinski, 2009).
Evaluating Social Forecasting vs. Traditional Forecasting
The Social Forecasting process was launched along the existing forecasting process for new products to allow for a direct comparison of the performance of both approaches. When the actual sales figures came in the project team computed the forecasting accuracy of both approaches. Table 1 shows the forecasting accuracy from both approaches. The established new product evaluation process had a forecasting accuracy of 87% while the new Social Forecasting process achieved 81% in its first five weeks (Preller and Rudzinski, 2009).
social forecasting | existing process | |
---|---|---|
forecasting accuracy | 81% | 87% |
cost per new product | 90% lower | several thousand Euro |
time required | 1 week | 4 weeks |
Table 1: Comparison of forecasting accuracy for new products (Source: Author)
The accuracy of the new approach is lower. A deeper look at the data on Fig. 4 reveals that most products have a moderate share of 1% or less in the total sales. These low-selling products are slightly better forecasted by Tchibo existing forecasting procedure. Only a few products are able to capture more than 1% of total sales. Those high-selling products are better forecasted by Social Forecasting. When computing the unweighted average forecasting accuracy, the existing forecasting approach is more accurate as seen in the previous table.
Fig. 4: Forecasting accuracy by product by share in sales (Source: Author)
Another advantage of Social forecasting was its speed. Collecting the knowledge from employees required only about 1 week while results from surveys and test sales came in only after 4 weeks. Tests had to be launched well in advance in order to be available on time. Social Forecasting added significant flexibility here.
From a single product-perspective the cost of evaluating a new product by Social Forecasting were 90% lower than those of the existing forecasting process. This extreme cost shift is possible because drawing on the existing knowledge of employees is practically for free, while the cost of representative surveys and test sales is quite high.
Conclusion
Forecasting new products is hard. There is no past data to use in statistical models, consumer surveys and test sales are expensive. In such a situation a Collective Intelligence-based approach like Social Forecasting was able to provide high-quality forecasts at extremely low cost.
The performance of Social Forecasting was well received by the project team and warranted the regular use of Social Forecasting at Tchibo. This new forecasting process would not completely replace traditional market research and test sales but only part of them in order to combine the strengths of both approaches while saving forecasting costs on less important products. Tchibo decided to use Social Forecasting primarily on its high-selling products. These products required the same forecasting effort as any other product but had a much higher ROI on forecasting accuracy.
The total annual cost savings from replacing part of the surveys and test sales with Social Forecasting were in the range of single-digit million Euro figures. The additional EBIT benefits from higher forecasting accuracy are not discloseable.
An added bonus of Social Forecasting was less tangible: Shop managers and warehouse workers were very happy. The feeling of one’s own opinion mattering to HQ was boosting staff morale and triggered even more feedback and new product ideas from employees. While these are quite remarkable side effects they are beyond the scope of this case study which focused on the measurable benefits of sourcing employee knowledge with a new and innovative approach.
References
Armstrong, J.Scott (2001): Principles of Forecasting, Springer Press, New York, p. 682
Ivanov, A. (2011): Sales Forecasts without Market Research: How does it work?, Controller Magazin, September/October 2011
Preller, G; Rudzinski, C. (2009): Ich sehe was, was du nicht siehst: Informationsmärkte analysiert anhand eines filialisierten Einzelhändlers, in Aulinger, A.; Pfeiffer, M (ed.) (2009): Kollektive Intelligenz. Methoden, Erfahrungen und Perspektiven, Steinbeis-Edition, Stuttgart/Berlin, p. 151-176
Kommentare
Das Kommentarsystem ist zurzeit deaktiviert.
Themengruppen
Dieser Beitrag ist den folgenden Themengruppen zugeordnet
Schlagworte
Dieser Beitrag ist den folgenden Schlagworten zugeordnet